In the dynamic landscape of technology and innovation, data science has emerged as a powerhouse, revolutionizing industries across the globe. In India, the demand for skilled data scientists is soaring, leading to a surge in the availability of data science course in India. As individuals seek to capitalize on this trend, it becomes crucial to navigate the diverse array of options available in the country.
Data Science Course in India: A Multifaceted Journey
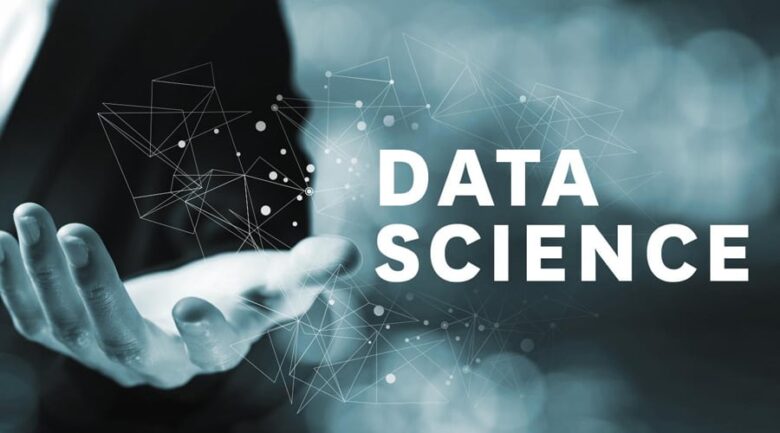
Embarking on a career in data science begins with choosing the right educational path. The plethora of data science courses in India can be both exciting and overwhelming. From traditional university programs to specialized online courses, aspiring data scientists have a myriad of options to consider. The curriculum typically covers statistical analysis, machine learning, data visualization, and programming languages such as Python and R.
Leading educational institutions and online platforms offer comprehensive courses tailored to meet the industry’s evolving demands. Institutions like the Indian Institutes of Technology (IITs), Indian Institutes of Management (IIMs), and renowned online platforms like Coursera and edX provide diverse programs to suit various learning preferences.
Explore Data Science Pay After Placement Programs
In recent years, a novel approach has gained popularity among those aspiring to pursue data science courses – the pay after placement model. This innovative program structure allows students to enroll in a data science course without the burden of hefty upfront fees. Instead, learners pay the course fees only after securing a job in the field.
This pay after placement model not only makes quality education accessible but also aligns the interests of educational institutions with the success of their students. As the industry seeks skilled professionals, this approach fosters a symbiotic relationship between academia and the workforce.
Aspiring data scientists can explore various Explore data science pay after placement programs offered by reputed institutions and online platforms. These programs often include career support services, mentorship opportunities, and industry connections to enhance the chances of successful placement upon completion of the course.
Is Data Scientist a Good Career?
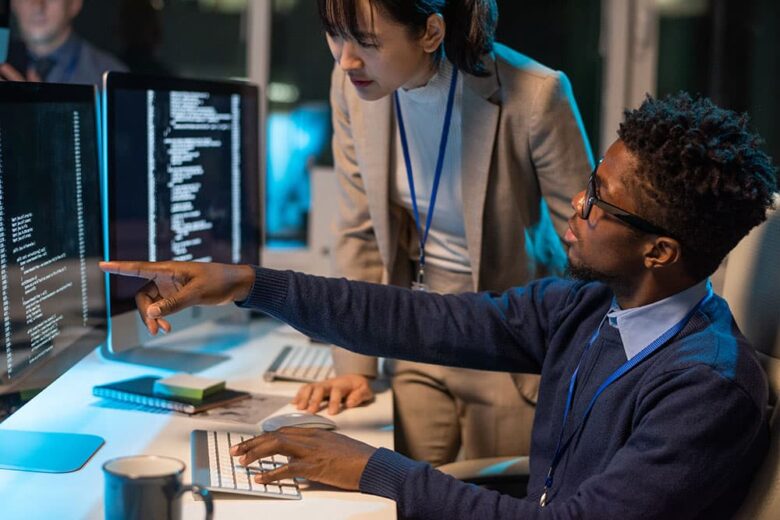
Amidst the growing interest in data science courses, the question on many minds Is data scientist a good career? The answer is a resounding yes. The role of a data scientist is not only lucrative but also pivotal in today’s data-driven world. Organizations across industries rely on data scientists to extract valuable insights from vast datasets, driving informed decision-making.
Data scientists are in high demand, and this trend is expected to persist as industries continue to embrace digital transformation. The diverse applications of data science, from healthcare to finance, make it a versatile and future-proof career choice.
The Essence of Data Science:
At its core, data science is the art and science of transforming raw data into actionable insights. It encompasses a wide array of techniques, methodologies, and tools to dissect complex datasets, discover trends, and predict future outcomes. From business analytics to healthcare informatics, data science finds applications in diverse domains, empowering organizations to make strategic decisions based on evidence rather than intuition.
Key Components of Data Science:
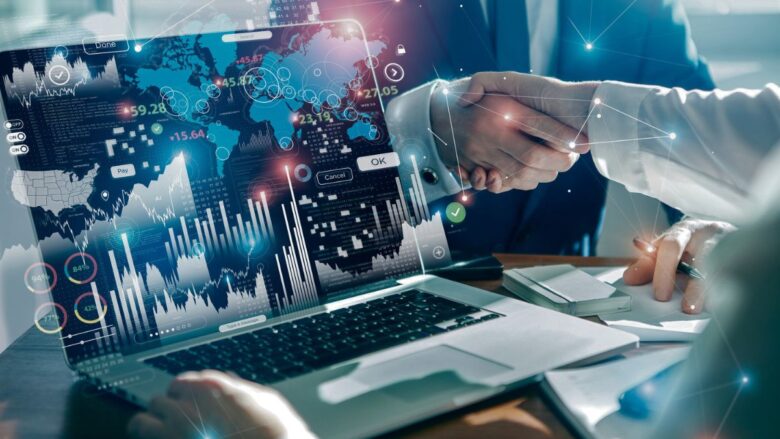
Data Collection and Cleaning:
Data scientists begin by collecting raw data from various sources, such as sensors, databases, or APIs. However, raw data is often messy and unstructured. Cleaning and preprocessing are crucial steps to ensure the quality and reliability of the data before analysis.
Exploratory Data Analysis (EDA):
EDA involves visually and statistically exploring datasets to uncover patterns, anomalies, and relationships. This phase sets the stage for further analysis and helps data scientists gain a deeper understanding of the data.
Feature Engineering:
Feature engineering involves selecting, transforming, or creating new features to enhance the performance of machine learning models. This step requires a deep understanding of both the data and the problem at hand.
Machine Learning:
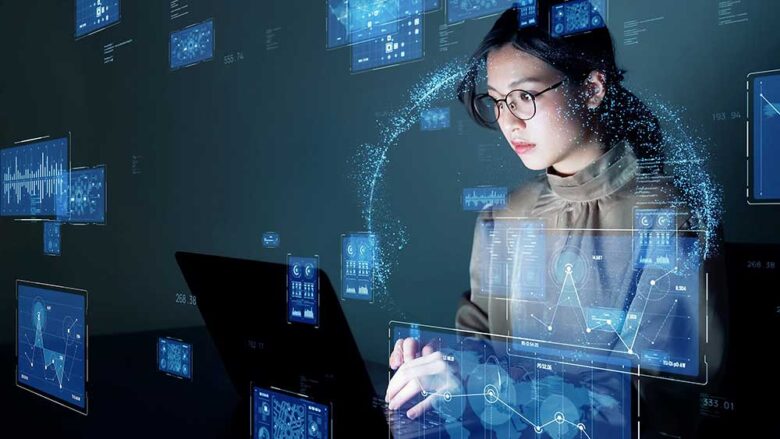
Machine learning algorithms are the heart of data science. These algorithms learn from historical data and make predictions or decisions without being explicitly programmed. Supervised learning, unsupervised learning, and reinforcement learning are common paradigms within machine learning.
Model Evaluation and Validation:
After training a model, it is essential to evaluate its performance using validation datasets. This step ensures that the model generalizes well to new, unseen data and provides reliable predictions.
Deployment and Monitoring:
Successful models are deployed into production environments where they can provide real-time insights. Continuous monitoring is crucial to ensure that the model maintains its accuracy as new data becomes available.
Impact of Data Science:
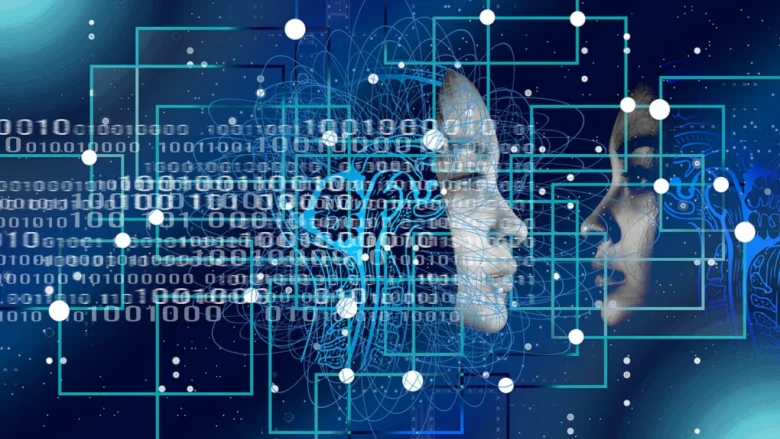
Business Intelligence:
Data science empowers businesses to gain a competitive edge by extracting valuable insights from customer behavior, market trends, and operational efficiency. This information aids in strategic decision-making, product development, and targeted marketing.
Healthcare Advancements:
In healthcare, data science facilitates disease prediction, personalized medicine, and clinical decision support. Analyzing patient data can lead to early diagnosis and improved treatment outcomes.
Financial Modeling:
The financial sector leverages data science for risk management, fraud detection, and algorithmic trading. Predictive models help investors make informed decisions, minimizing uncertainties in the volatile financial landscape.
Smart Cities and IoT:
Data science plays a pivotal role in creating smart cities by analyzing data from sensors, cameras, and other IoT devices. This enables efficient urban planning, traffic management, and resource optimization.
Conclusion:
Data science is more than a buzzword; it is a transformative force shaping the future of industries and societies. As the volume of data continues to grow, the demand for skilled data scientists will only intensify. In the pursuit of innovation and progress, organizations must harness the power of data science to unlock new possibilities, drive informed decision-making, and stay ahead in an ever-evolving digital landscape.